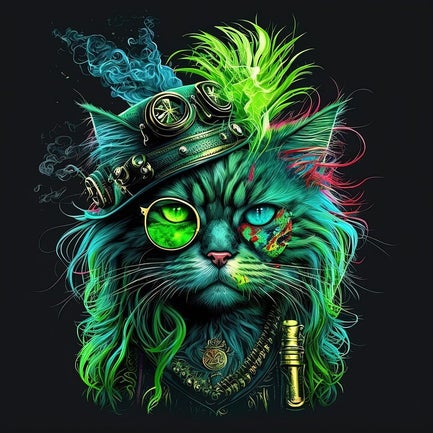
Technology is one of the most influential forces in history but also today. Nowadays, technological progress occurs in networks. Artificial Intelligence (AI), connectivity and digitalization, all three terms describe the same phenomenon from different perspectives. Let's have a look at the different dimensions.
Artificial intelligence (AI) is the generic term for applications in which machines perform human-like intelligence services such as learning, judgment and problem solving. It is being used to forecast, automate, and optimize human tasks, such as speech and facial recognition, decision making, and translation. These abilities are learned with the help of machine learning (ML) and deep learning (DL). Neural networks build the basis for these types of 'learning’. ML and DL differ in the number of node layers, or depth, of neural networks that are used. A deep learning algorithm operates more than three node layers.[1]
ML is more depending on human interventions when learning. This means that more structured data must be predetermined. This way, ML can automatically generate knowledge, train algorithms, identify correlations and recognize unknown patterns. ML predicts and optimizes processes of different kind. The ‘deep’ in DL performs a step further and processes complex data using multilayer neural networks. These continuously interlink what it has been learning at each level with new content. This way, DL can use unstructured data in its raw form (e. g. text, images) to recognize complex patterns and relationships in the data.
This enables the machine to forecast or make decisions and to question them. This is a huge step in significantly augmenting human capabilities and productivity with the help of artificial intelligence.
This was the report ROM_03 – Artificial Intelligence. We analyze megatrends, external disruptions, and internal organizational processes for their impacts on business risks, but also opportunities. The intention is to give impulses making companies fit for the future.
You are invited to participate in the discussion and contribute your experiences and examples. In doing so, you will be helping to expand and improve the knowledge about risk and opportunity management.
Deep learning applications are used in manifold industries:[2]
- Automotive industry: DL is a prerequisite for automated driving. It automatically recognizes objects such as stop signs and traffic lights. DL assists to detect pedestrians to avoid accidents.
- Aerospace and defense: DL facilitates identifying objects via satellites. This way appropriate areas for special applications can be recognized. It is also possible to identify safe or dangerous zones for emergency forces.
- Medical research: Cancer scientists use DL to automatically detect cancer cells. For this purpose, they use an innovative microscope which provides a high-dimensional data set. Based on this data set a specific DL application is able to accurately identify cancer cells.
- Industrial automation: DL helps to increase work safety on machinery by automatically detecting whether people or objects are dangerously close to machines.
- Electronics: Deep Learning is used for automated listening and speaking. For example, smart communication used in the private sphere is based on DL applications.
Additionally, edge computing, i.e. using decentralized server and data tools will let devices act independently from clouds and substitute to some extent human decision making and actions. Further examples are:[i]
- Virtual assistants on internet pages
- Digital image recognition
- Optimization of traffic control
- Calculation of optimal maintenance times
The next surge of technological development is already visible. 5G technology will increase data speed at 100 times and will allow millions of connected devices to continuously communicate with each other.
Another technology with huge potential hides behind quantum computing. It is a fundamentally different approach to computation than the kind of computations that today's computers perform. By leveraging the principles of quantum physics, quantum computers will be able to solve specific, typically very complex problems of a statistical nature that are too difficult for today's computers.
Risks & Opportunities & Recommendations
The above explained examples make it clear. AI, here especially DL, will more and more influence our business, but also private life. DL can be used to optimize production processes, the quality of products, the communication and so on. Companies need to be aware of the short-term, but also strategical changes.
Risks: Competition between companies for the effective use of AI is increasing. It is about optimizing production processes and developing new generations of products. Any company that does not take on these challenges will disappear from the market. It is expected that the use of AI in the workplace will lead to job savings. This will make companies think more about how to redesign production processes and which employees with which qualifications will be needed in the future. Education and training are facing huge challenges.
Opportunities: AI can help optimize distribution channels, improve maintenance techniques, increase production performance and quality, improve customer service and help save energy. This will concern all industries from green economy and recycling economy, to engineering, agriculture, healthcare, fashion and tourism.
The described technologies and further more step by step force companies to reshape their operations - and also to convert them. To the extent they do so, higher productivity will follow and new business models will be created.
Recommendations: C-suite should implement a task force that continuously analyses the company’s own products, services and processes to what extent these can be improved and optimized through AI applications. The results must then be strategically considered and implemented.
[1] See also AI vs. Machine Learning vs. Deep Learning vs. Neural Networks: What’s the Difference? | IBM
[2] For more information: Deep Learning: Drei Dinge, die Sie wissen sollten - MATLAB & Simulink (mathworks.com)
[i] Seven examples of artificial intelligence in everyday life, Handelsblatt, 22.3.2019.
Add comment
Comments